A New Age of Data Means Embracing the Edge
Business Lab - En podcast af MIT Technology Review Insights
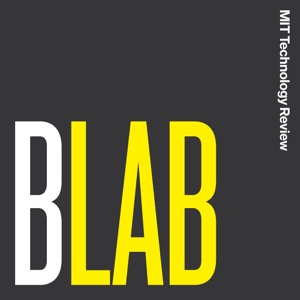
Artificial intelligence holds an enormous promise, but to be effective, it must learn from massive sets of data—and the more diverse the better. By learning patterns, AI tools can uncover insights and help decision-making not just in technology, but also pharmaceuticals, medicine, manufacturing, and more. However, data can’t always be shared—whether it’s personally identifiable, holds proprietary information, or to do so would be a security concern—until now. “It’s going to be a new age.” Says Dr. Eng Lim Goh, senior vice president and CTO of artificial intelligence at Hewlett Packard Enterprise. “The world will shift from one where you have centralized data, what we've been used to for decades, to one where you have to be comfortable with data being everywhere.” Data everywhere means the edge, where each device, server, and cloud instance collect massive amounts of data. One estimate has the number of connected devices at the edge increasing to 50 billion by 2022. The conundrum: how to keep collected data secure but also be able to share learnings from the data, which, in turn, helps teach AI to be smarter. Enter swarm learning. Swarm learning, or swarm intelligence, is how swarms of bees or birds move in response to their environment. When applied to data Goh explains, there is “more peer-to-peer communications, more peer-to-peer collaboration, more peer-to-peer learning.” And Goh continues, “That's the reason why swarm learning will become more and more important as …as the center of gravity shifts” from centralized to decentralized data. Consider this example, says Goh. “A hospital trains their machine learning models on chest X-rays and sees a lot of tuberculosis cases, but very little of lung collapsed cases. So therefore, this neural network model, when trained, will be very sensitive to what's detecting tuberculosis and less sensitive towards detecting lung collapse.” Goh continues, “However, we get the converse of it in another hospital. So what you really want is to have these two hospitals combine their data so that the resulting neural network model can predict both situations better. But since you can't share that data, swarm learning comes in to help reduce that bias of both the hospitals.” And this means, “each hospital is able to predict outcomes, with accuracy and with reduced bias, as though you have collected all the patient data globally in one place and learned from it,” says Goh. And it’s not just hospital and patient data that must be kept secure. Goh emphasizes “What swarm learning does is to try to avoid that sharing of data, or totally prevent the sharing of data, to [a model] where you only share the insights, you share the learnings. And that's why it is fundamentally more secure.”