Using Machine Learning to Build Maps That Give Smarter Driving Advice
Business Lab - En podcast af MIT Technology Review Insights
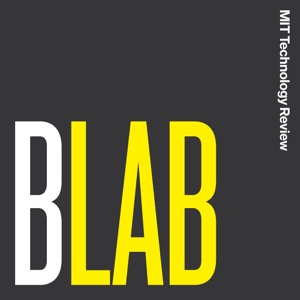
If you drive in the United States, chances are you can’t remember the last time you bought a paper map, printed out a digital map, or even stopped to ask for directions. Thanks to GPS and the mobile mapping apps on our smartphones and their real-time routing advice, navigation is a solved problem. But in developing or fast-growing parts of the world: not so much. If you live in a place like Doha, Qatar, where the length of the road network has tripled over the last five years, commercial mapping services from Google, Apple, Bing, or other providers simply can’t keep up with the pace of infrastructure change. “Each one of us who grew up in Europe or the US probably cannot understand the scale at which these cities grow,” says Rade Stanojevic, a senior scientist at the Qatar Computing Research Institute (QCRI), part of Hamad Bin Khalifa University, a Qatar Foundation university, in Doha. “Pretty much every neighborhood sees a new underpass, new overpass, new large highway being added every couple of months.” As Qatar copes with this rapid growth—and especially as it prepares to host the FIFA World Cup in 2022—the bad routing advice and accumulating travel delays from outdated digital maps is increasingly costly. That’s why Stanojevic and colleagues at QCRI decided to try applying machine learning to the problem. A road network can be interpreted as a giant graph with where every intersection is a node and every road is an edge, says Stanojevic, whose specialty is network economics. Road segments can have both static characteristics, such as the designated speed limit, and dynamic characteristics, such as rush-hour congestion. To see where traffic really is going—rather than where an old map says it should go—and then predict the best routes through an ever-changing maze, all a machine-learning model would need is lots of up-to-data data on both the static and dynamic factors. “Fortunately enough, modern vehicle fleets have these monitoring systems that produce quite a lot of data,” says Stanojevic. Stanojevic is talking about taxis. His team at QCRI partnered with a Doha-based taxi company called Karwa to collect full GPS data on their vehicles’ comings and goings. They used that data to build a new mapping service called QARTA that offers routing advice to drivers at Karwa and other operators such as delivery fleets. Stanojevic says QARTA’s deeper understanding of the actual road and traffic situation in Doha helps drivers shave tens of seconds off every trip, which translates into a fleet-wide efficiency gain of 5 to 10 percent. “If you’re running a fleet of 3,000 cars, five percent of that is 150 cars,” Stanojevic says. “You can basically remove 150 cars from the road and not lose any business.” Although QCRI’s system probably can’t compete with the big map-services providers in the developed world, it could help cities in the Middle East and other developing regions manage growth more wisely, Stanojevic says. And a few years from now, as more autonomous vehicles take to the streets, machine-learning-based routing advice could look at the big picture in a busy city and help fleets cut carbon emissions by keeping drivers out of traffic jams. “By having some sort of a global view of what’s going on in the whole city, autonomous vehicles can actually reroute us to have some sort of global load balancing, to help everyone be better off.” This podcast was produced in partnership with the Qatar Foundation. Show notes and links Qatar Computing Research Institute Sofiane Abbar, Rade Stanojevic, Shadab Mustafa, and Mohamed Mokbel, Traffic Routing in the Ever-Changing City of Doha, Communications of the ACM, April 2021