Data Observability - Barr Moses
DataTalks.Club - En podcast af DataTalks.Club
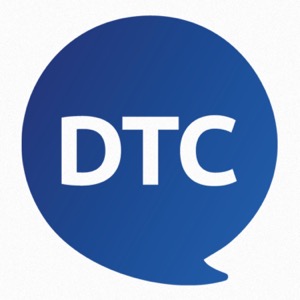
Kategorier:
We covered: Barr’s background Market gaps in data reliability Observability in engineering Data downtime Data quality problems and the five pillars of data observability Example: job failing because of a schema change Three pillars of observability (good pipelines and bad data) Observability vs monitoring Finding the root cause Who is accountable for data quality? (the RACI framework) Service level agreements Inferring the SLAs from the historical data Implementing data observability Data downtime maturity curve Monte carlo: data observability solution Open source tools Test-driven development for data Is data observability cloud agnostic? Centralizing data observability Detecting downstream and upstream data usage Getting bad data vs getting unusual data Links: Learn more about Monte Carlo: https://www.montecarlodata.com/ The Data Engineer's Guide to Root Cause Analysis: https://www.montecarlodata.com/the-data-engineers-guide-to-root-cause-analysis/ Why You Need to Set SLAs for Your Data Pipelines: https://www.montecarlodata.com/how-to-make-your-data-pipelines-more-reliable-with-slas/ Data Observability: The Next Frontier of Data Engineering: https://www.montecarlodata.com/data-observability-the-next-frontier-of-data-engineering/ To get in touch with Barr, ping her in the DataTalks.Club group or use [email protected] Join DataTalks.Club: https://datatalks.club/slack.html