DataOps, Observability, and The Cure for Data Team Blues - Christopher Bergh
DataTalks.Club - En podcast af DataTalks.Club - Fredage
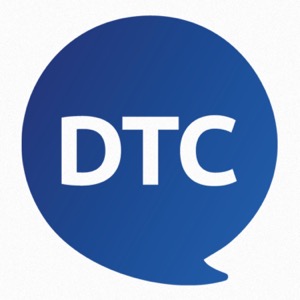
Kategorier:
0:00 hi everyone Welcome to our event this event is brought to you by data dos club which is a community of people who love 0:06 data and we have weekly events and today one is one of such events and I guess we 0:12 are also a community of people who like to wake up early if you're from the states right Christopher or maybe not so 0:19 much because this is the time we usually have uh uh our events uh for our guests 0:27 and presenters from the states we usually do it in the evening of Berlin time but yes unfortunately it kind of 0:34 slipped my mind but anyways we have a lot of events you can check them in the 0:41 description like there's a link um I don't think there are a lot of them right now on that link but we will be 0:48 adding more and more I think we have like five or six uh interviews scheduled so um keep an eye on that do not forget 0:56 to subscribe to our YouTube channel this way you will get notified about all our future streams that will be as awesome 1:02 as the one today and of course very important do not forget to join our community where you can hang out with 1:09 other data enthusiasts during today's interview you can ask any question there's a pin Link in live chat so click 1:18 on that link ask your question and we will be covering these questions during the interview now I will stop sharing my 1:27 screen and uh there is there's a a message in uh and Christopher is from 1:34 you so we actually have this on YouTube but so they have not seen what you wrote 1:39 but there is a message from to anyone who's watching this right now from Christopher saying hello everyone can I 1:46 call you Chris or you okay I should go I should uh I should look on YouTube then okay yeah but anyways I'll you don't 1:53 need like you we'll need to focus on answering questions and I'll keep an eye 1:58 I'll be keeping an eye on all the question questions so um 2:04 yeah if you're ready we can start I'm ready yeah and you prefer Christopher 2:10 not Chris right Chris is fine Chris is fine it's a bit shorter um 2:18 okay so this week we'll talk about data Ops again maybe it's a tradition that we talk about data Ops every like once per 2:25 year but we actually skipped one year so because we did not have we haven't had 2:31 Chris for some time so today we have a very special guest Christopher Christopher is the co-founder CEO and 2:37 head chef or hat cook at data kitchen with 25 years of experience maybe this 2:43 is outdated uh cuz probably now you have more and maybe you stopped counting I 2:48 don't know but like with tons of years of experience in analytics and software engineering Christopher is known as the 2:55 co-author of the data Ops cookbook and data Ops Manifesto and it's not the 3:00 first time we have Christopher here on the podcast we interviewed him two years ago also about data Ops and this one 3:07 will be about data hops so we'll catch up and see what actually changed in in 3:13 these two years and yeah so welcome to the interview well thank you for having 3:19 me I'm I'm happy to be here and talking all things related to data Ops and why 3:24 why why bother with data Ops and happy to talk about the company or or what's changed 3:30 excited yeah so let's dive in so the questions for today's interview are prepared by Johanna berer as always 3:37 thanks Johanna for your help so before we start with our main topic for today 3:42 data Ops uh let's start with your ground can you tell us about your career Journey so far and also for those who 3:50 have not heard have not listened to the previous podcast maybe you can um talk 3:55 about yourself and also for those who did listen to the previous you can also maybe give a summary of what has changed 4:03 in the last two years so we'll do yeah so um my name is Chris so I guess I'm 4:09 a sort of an engineer so I spent about the first 15 years of my career in 4:15 software sort of working and building some AI systems some non- AI systems uh 4:21 at uh Us's NASA and MIT linol lab and then some startups and then um 4:30 Microsoft and then about 2005 I got I got the data bug uh I think you know my 4:35 kids were small and I thought oh this data thing was easy and I'd be able to go home uh for dinner at 5 and life 4:41 would be fine um because I was a big you started your own company right and uh it didn't work out that way 4:50 and um and what was interesting is is for me it the problem wasn't doing the 4:57 data like I we had smart people who did data science and data engineering the act of creating things it was like the 5:04 systems around the data that were hard um things it was really hard to not have 5:11 errors in production and I would sort of driving to work and I had a Blackberry at the time and I would not look at my 5:18 Blackberry all all morning I had this long drive to work and I'd sit in the parking lot and take a deep breath and 5:24 look at my Blackberry and go uh oh is there going to be any problems today and I'd be and if there wasn't I'd walk and 5:30 very happy um and if there was I'd have to like rce myself um and you know and 5:36 then the second problem is the team I worked for we just couldn't go fast enough the customers were super 5:42 demanding they didn't care they all they always thought things should be faster and we are always behind and so um how 5:50 do you you know how do you live in that world where things are breaking left and right you're terrified of making errors 5:57 um and then second you just can't go fast enough um and it's preh Hadoop era 6:02 right it's like before all this big data Tech yeah before this was we were using 6:08 uh SQL Server um and we actually you know we had smart people so we we we 6:14 built an engine in SQL Server that made SQL Server a column or 6:20 database so we built a column or database inside of SQL Server um so uh 6:26 in order to make certain things fast and and uh yeah it was it was really uh it's not 6:33 bad I mean the principles are the same right before Hadoop it's it's still a database there's still indexes there's 6:38 still queries um things like that we we uh at the time uh you would use olap 6:43 engines we didn't use those but you those reports you know are for models it's it's not that different um you know 6:50 we had a rack of servers instead of the cloud um so yeah and I think so what what I 6:57 took from that was uh it's just hard to run a team of people to do do data and analytics and it's not 7:05 really I I took it from a manager perspective I started to read Deming and 7:11 think about the work that we do as a factory you know and in a factory that produces insight and not automobiles um 7:18 and so how do you run that factory so it produces things that are good of good 7:24 quality and then second since I had come from software I've been very influenced 7:29 by by the devops movement how you automate deployment how you run in an agile way how you 7:35 produce um how you how you change things quickly and how you innovate and so 7:41 those two things of like running you know running a really good solid production line that has very low errors 7:47 um and then second changing that production line at at very very often they're kind of opposite right um and so 7:55 how do you how do you as a manager how do you technically approach that and 8:00 then um 10 years ago when we started data kitchen um we've always been a profitable company and so we started off 8:07 uh with some customers we started building some software and realized that we couldn't work any other way and that 8:13 the way we work wasn't understood by a lot of people so we had to write a book and a Manifesto to kind of share our our 8:21 methods and then so yeah we've been in so we've been in business now about a little over 10 8:28 years oh that's cool and uh like what 8:33 uh so let's talk about dat offs and you mentioned devops and how you were inspired by that and by the way like do 8:41 you remember roughly when devops as I think started to appear like when did people start calling these principles 8:49 and like tools around them as de yeah so agile Manifesto well first of all the I 8:57 mean I had a boss in 1990 at Nasa who had this idea build a 9:03 little test a little learn a lot right that was his Mantra and then which made 9:09 made a lot of sense um and so and then the sort of agile software Manifesto 9:14 came out which is very similar in 2001 and then um the sort of first real 9:22 devops was a guy at Twitter started to do automat automated deployment you know 9:27 push a button and that was like 200 Nish and so the first I think devops 9:33 Meetup was around then so it's it's it's been 15 years I guess 6 like I was 9:39 trying to so I started my career in 2010 so I my first job was a Java 9:44 developer and like I remember for some things like we would just uh SFTP to the 9:52 machine and then put the jar archive there and then like keep our fingers crossed that it doesn't break uh uh like 10:00 it was not really the I wouldn't call it this way right you were deploying you 10:06 had a Dey process I put it yeah 10:11 right was that so that was documented too it was like put the jar on production cross your 10:17 fingers I think there was uh like a page on uh some internal Viki uh yeah that 10:25 describes like with passwords and don't like what you should do yeah that was and and I think what's interesting is 10:33 why that changed right and and we laugh at it now but that was why didn't you 10:38 invest in automating deployment or a whole bunch of automated regression 10:44 tests right that would run because I think in software now that would be rare 10:49 that people wouldn't use C CD they wouldn't have some automated tests you know functional 10:56 regression tests that would be the exception whereas that the norm at the beginning of your career and so that's 11:03 what's interesting and I think you know if we if we talk about what's changed in the last two three years I I think it is 11:10 getting more standard there are um there's a lot more companies who are 11:15 talking data Ops or data observability um there's a lot more tools that are a lot more people are 11:22 using get in data and analytics than ever before I think thanks to DBT um and 11:29 there's a lot of tools that are I think getting more code Centric right that 11:35 they're not treating their configuration like a black box there there's several 11:41 bi tools that tout the fact that they that they're uh you know they're they're git Centric you know and and so and that 11:49 they're testable and that they have apis so things like that I think people maybe let's take a step back and just do a 11:57 quick summary of what data Ops data Ops is and then we can talk about like what changed in the last two years sure so I 12:06 guess it starts with a problem and that it's it sort of 12:11 admits some dark things about data and analytics and that we're not really successful and we're not really happy um 12:19 and if you look at the statistics on sort of projects and problems and even 12:25 the psychology like I think about a year or two we did a survey of 12:31 data Engineers 700 data engineers and 78% of them wanted their job to come with a therapist and 50% were thinking 12:38 of leaving the career altogether and so why why is everyone sort of unhappy well I I I think what happens is 12:46 teams either fall into two buckets they're sort of heroic teams who 12:52 are doing their they're working night and day they're trying really hard for their customer um and then they get 13:01 burnt out and then they quit honestly and then the second team have wrapped 13:06 their projects up in so much process and proceduralism and steps that doing 13:12 anything is sort of so slow and boring that they again leave in frustration um 13:18 or or live in cynicism and and that like the only outcome is quit and 13:24 start uh woodworking yeah the only outcome really is quit and start working 13:29 and um as a as a manager I always hated that right because when when your team 13:35 is either full of heroes or proceduralism you always have people who have the whole system in their head 13:42 they're certainly key people and then when they leave they take all that knowledge with them and then that 13:48 creates a bottleneck and so both of which are aren aren't and I think the 13:53 main idea of data Ops is there's a balance between fear and herois 14:00 that you can live you don't you know you don't have to be fearful 95% of the time maybe one or two% it's good to be 14:06 fearful and you don't have to be a hero again maybe one or two per it's good to be a hero but there's a balance um and 14:13 and in that balance you actually are much more prod