The Shipyard: Lessons Learned While Building an ML Platform / Automating Adherence // Joseph Haaga // Coffee Sessions #91
MLOps.community - En podcast af Demetrios
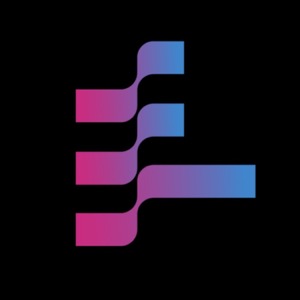
Kategorier:
MLOps Coffee Sessions #91 with Joseph Haaga, The Shipyard: Lessons Learned While Building an ML Platform / Automating Adherence. // Abstract Joseph Haaga and the Interos team walk us through their design decisions in building an internal data platform. Joseph talks about why their use case wasn't a fit for off the self solutions, what their internal tool snitch does, and how they use git as a model registry. Shipyard blogpost series: https://medium.com/interos-engineering. // Bio Joseph leads the ML Platform team at Interos, the operational resilience company. He was introduced to ML Ops while working as a Senior Data Engineer and has spent the past year building a platform for experimentation and serving. He lives in Washington, DC, with his dog Cheese. // MLOps Jobs board https://mlops.pallet.xyz/jobs // Related Links Website: https://joehaaga.xyz Medium: https://medium.com/interos-engineering Shipyard blogpost series: https://medium.com/interos-engineering --------------- ✌️Connect With Us ✌️ ------------- Join our slack community: https://go.mlops.community/slack Follow us on Twitter: @mlopscommunity Sign up for the next meetup: https://go.mlops.community/register Catch all episodes, blogs, newsletters, and more: https://mlops.community/ Connect with Demetrios on LinkedIn: https://www.linkedin.com/in/dpbrinkm/ Connect with Vishnu on LinkedIn: https://www.linkedin.com/in/vrachakonda/ Connect with Joseph on LinkedIn: https://www.linkedin.com/in/joseph-haaga/ Timestamps: [00:00] Introduction to Joseph Haaga [02:07] Please subscribe, follow, like, rate, review our Spotify and Youtube channels [02:31] New! Best of Slack Weekly Newsletter [03:03] Interos [04:33] Global supply chain [05:45] Machine Learning use cases of Interos [06:17] Forecasting and optimization of routes [07:14] Build, buy, open-source decision making [10:06] Experiences with Kubeflow [11:05] Creating standards and rules when creating the platform [13:29] Snitches [14:10] Inter-team discussions when processes fall apart [16:56] Examples of the development process on the feedback of ML engineers and data scientists [20:35] Preserving flexibility when introducing new models and formats [21:37] Organizational structure of Interos [23:40] Surface area for product [24:46] Use of Git Ops to manage boarding pass [28:04] Cultural emphasis [30:02] Naming conventions [32:28] Benefit of a clean slate [33:16] One-size-fits-all choice [37:34] Wrap up