Preetum Nakkiran: An Empirical Theory of Deep Learning
The Gradient: Perspectives on AI - En podcast af Daniel Bashir
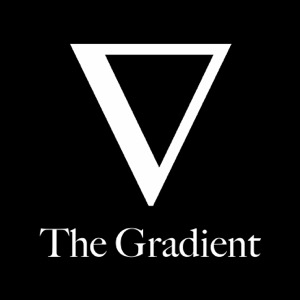
Kategorier:
In episode 31 of The Gradient Podcast, Daniel Bashir speaks to Preetum Nakkiran.Preetum is a Research Scientist at Apple, a Visiting Researcher at UCSD, and part of the NSF/Simons Collaboration on the Theoretical Foundations of Deep Learning. He completed his PhD at Harvard, where he co-founded the ML Foundations Group. Preetum’s research focuses on building conceptual tools for understanding learning systems.Subscribe to The Gradient Podcast: Apple Podcasts | Spotify | Pocket Casts | RSSFollow The Gradient on TwitterSections:(00:00) Intro(01:25) Getting into AI through Theoretical Computer Science (TCS)(09:08) Lack of Motivation in TCS and Learning What Research Is(12:12) Foundational vs Problem-Solving Research, Antipatterns in TCS(16:30) Theory and Empirics in Deep Learning(18:30) What is an Empirical Theory of Deep Learning(28:21) Deep Double Descent(40:00) Inductive Biases in SGD, epoch-wise double descent(45:25) Inductive Biases Stick Around(47:12) Deep Bootstrap(59:40) Distributional Generalization - Paper Rejections(1:02:30) Classical Generalization and Distributional Generalization(1:16:46) Future Work: Studying Structure in Data(1:20:51) The Tweets^TM(1:37:00) OutroEpisode Links:* Preetum’s Homepage* Preetum’s PhD Thesis Get full access to The Gradient at thegradientpub.substack.com/subscribe