85. Brian Christian - The Alignment Problem
Towards Data Science - En podcast af The TDS team
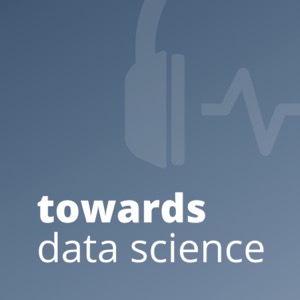
Kategorier:
In 2016, OpenAI published a blog describing the results of one of their AI safety experiments. In it, they describe how an AI that was trained to maximize its score in a boat racing game ended up discovering a strange hack: rather than completing the race circuit as fast as it could, the AI learned that it could rack up an essentially unlimited number of bonus points by looping around a series of targets, in a process that required it to ram into obstacles, and even travel in the wrong direction through parts of the circuit. This is a great example of the alignment problem: if we’re not extremely careful, we risk training AIs that find dangerously creative ways to optimize whatever thing we tell them to optimize for. So building safe AIs — AIs that are aligned with our values — involves finding ways to very clearly and correctly quantify what we want our AIs to do. That may sound like a simple task, but it isn’t: humans have struggled for centuries to define “good” metrics for things like economic health or human flourishing, with very little success. Today’s episode of the podcast features Brian Christian — the bestselling author of several books related to the connection between humanity and computer science & AI. His most recent book, The Alignment Problem, explores the history of alignment research, and the technical and philosophical questions that we’ll have to answer if we’re ever going to safely outsource our reasoning to machines. Brian’s perspective on the alignment problem links together many of the themes we’ve explored on the podcast so far, from AI bias and ethics to existential risk from AI.